Christopher Mims, a tech columnist for The Wall Street Journal, argues that all businesses should operate like tech companies. This is especially apt for biotech companies focused on solving the grand challenges of our era. Transitioning into a Digital Biotech or TechBio enterprise allows for the agility seen in tech firms, characterized by a "Lab-as-Code" ethos and optimization for AI capabilities.
The TechBio vs. Conventional Biotech Divide
Traditional biotech endeavors often involve lengthy timelines and immense capital investments before impactful outcomes can be achieved. In contrast, companies like 23andMe and Moderna represent the TechBio model—leveraging cloud computing, data analytics, and AI to accelerate scaling and market entry. Key to TechBio operations is the "Lab-as-Code" paradigm, transforming lab processes into scalable, replicable units, similar to code repositories. Are you incorporating digital systems to enable a "Lab-as-Code" methodology?
Defining Digital Biotech/TechBio
TechBio, also known as Digital Biotech, aims to revolutionize the traditional biotech model by incorporating a 'digital twin' of your existing physical biotech infrastructure. This concept goes well beyond the adoption of advanced software solutions. It establishes an integrated ecosystem where automated data collection, real-time analytics, and AI-driven decision-making are the norms rather than the exceptions. Companies like Moderna exemplify this approach by using digital tools to manage vast pools of research data, thereby significantly accelerating vaccine development timelines. Similarly, Ginkgo Bioworks employs machine learning algorithms to optimize complex lab experiments, reducing the guesswork and increasing the throughput of valuable discoveries.
What sets TechBio companies apart is their enormous scalability, rooted in highly repeatable and predictable workflows. By utilizing advanced technologies like AI and machine learning, these companies substantially minimize variability in research processes. These digital tools enable the system to learn and improve with every new piece of data, making the entire operation more efficient, faster, and increasingly scalable over time. In essence, TechBio entities are evolutionary biotech companies with a self-improving, data-centric core. They are the epitome of scientific advancement and operational efficiency merged into one. So, are you prepared to leverage AI, machine learning, and integrated data repositories for instantaneous, actionable decision-making?
Advantages of the TechBio Transformation
Going TechBio has multiple operational benefits. Automation of tasks like data entry enables executives and researchers to focus on vital goals. For instance, Ginkgo Bioworks has automated many DNA sequencing steps, simplifying R&D operations. A fully digitized setup, like that at Moderna, offers a 360-degree view of your entire R&D pipeline. Moderna utilized its digital core to accelerate COVID-19 vaccine development, tracking real-time data on everything from molecular design to clinical trial results. This centralized view helped coordinate efforts across multiple departments, enabling faster decision-making. Are you reaching this level of automation and oversight with your existing setup?
Why You Should Aim for TechBio
If your startup is still relying on fragmented data across paper notebooks, emails, and siloed software systems like ELNs and LIMS, then you're not positioned for a TechBio transformation. Look to companies like Synthego, which leverages AI and cloud computing for CRISPR genome engineering, speeding up genetic research. Another example is Zymergen, which combines machine learning and genomics to optimize microbial factories for material production. These companies exemplify the TechBio approach, integrating AI and unified data systems for competitive advantage. Are you prepared to make the shift?
Key Steps to Evolve into a Digital Biotech/TechBio Enterprise
Adhere to ALCOA+ Principles: These principles stand for Attributable, Legible, Contemporaneous, Original, Accurate, Complete, Consistent, Enduring, and Available. Companies like Pfizer employ these principles rigorously in their clinical trial data management, utilizing digital platforms that offer electronic signatures, time-stamping, and secure storage. Is your data infrastructure prepared to meet these stringent ALCOA+ requirements?
Follow FAIR Data Protocols: This acronym stands for data that is Findable, Accessible, Interoperable, and Reusable. Research institutes like the European Molecular Biology Laboratory (EMBL) implement FAIR principles in their genomic databases, ensuring that their extensive data sets are easy to locate and reutilize. Is your data repository FAIR-compliant?
Automation and Integration: Companies such as Palantir Technologies and Ginkgo Bioworks have taken automation and integration to an advanced level. Palantir, for example, has collaborated with organizations like the National Institutes of Health to provide fully automated and integrated data analytics platforms. Ginkgo Bioworks has been streamlining DNA sequencing through automation, making high-throughput, complex experiments more manageable. Is your tech stack set up for this advanced level of automation and integration?
These key steps are foundational for any biotech company aiming to evolve into a TechBio enterprise. Make sure to evaluate and possibly restructure your data infrastructure and technological stack to align with these advanced protocols and systems.
Conclusion
Stepping into the Digital Biotech or TechBio arena isn't just chasing a trend; it's essential for maintaining competitiveness and impact in our increasingly data-centric world. This innovative model pairs a "Lab-as-Code" ethos with readiness for AI and data analytics. The risk of not adapting is clear: falling behind in a fast-paced industry. On the flip side, a successful transition can lead to exponential scaling, accelerated innovation, and expanded societal impact. Companies like Moderna and Ginkgo Bioworks offer compelling examples of leveraging TechBio for rapid vaccine development and lab optimization. Moreover, platforms like Scispot are democratizing this TechBio transformation, making it possible for any biotech company to operate as a TechBio and AI enterprise. If you're ready to adopt advanced strategies like ALCOA+ principles, FAIR data protocols, and sophisticated automation, book a consultation with experts in the field. Your present decisions will set the stage for meeting future challenges and seizing upcoming opportunities.
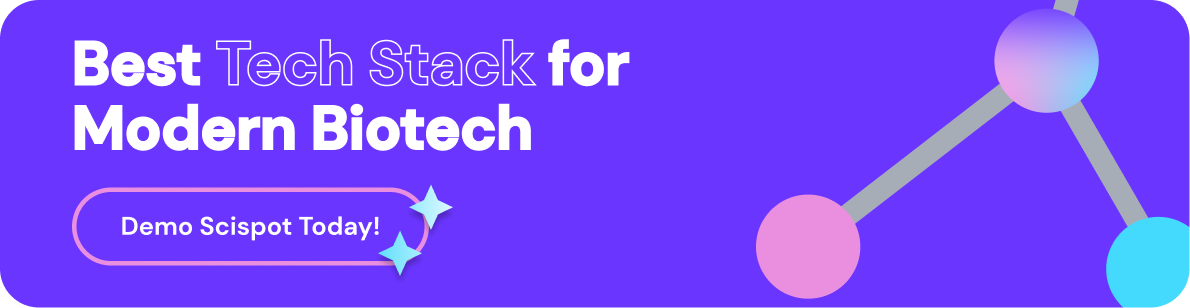