The bioinformatics team had the data. The proteomics researchers needed it. But between them was a tangled mess of spreadsheets, file formats, and disconnected systems. The manufacturing team, waiting on peptide designs, could do nothing but sit tight. Another production cycle was delayed—not by lack of scientific expertise, but by a broken workflow.
A synthetic peptide company in Boston was revolutionizing AI-driven peptide design for food supplements, but its internal processes were stuck in the past. Every hand-off meant lost time. Every misformatted dataset meant rework. And every delay in peptide manufacturing meant potential weeks lost in production cycles.
The Breaking Point: When Data Became a Roadblock
The company’s growth was outpacing its systems. Key challenges mounted:
- Data silos disrupted workflows – Manufacturing, proteomics, and bioinformatics teams worked in isolation, making hand-offs slow and error-prone.
- Lack of standardized LCMS templates – Inconsistent mass spectrometry data formats led to misinterpretations and delays.
- Disconnected experiment metadata – Critical data from mass spectrometry instruments wasn’t linked to experimental conditions, blocking meaningful insights.
- Manual process bottlenecks – Creating and tracking research requests between teams was cumbersome and time-consuming.
They tried piecing together custom scripts. They attempted manual workarounds. Nothing worked at scale. That’s when they realized they needed a system designed for AI-powered biotech—one that could connect their teams, not just store their data.
The Turning Point: A Data-First Mindset
The company needed more than just another data tool—it needed an operating system built for AI-powered biotech. That’s when they turned to Scispot OS. Instead of forcing teams to adapt to rigid software, Scispot adapted to them, integrating seamlessly into their workflows and breaking down the barriers between teams.
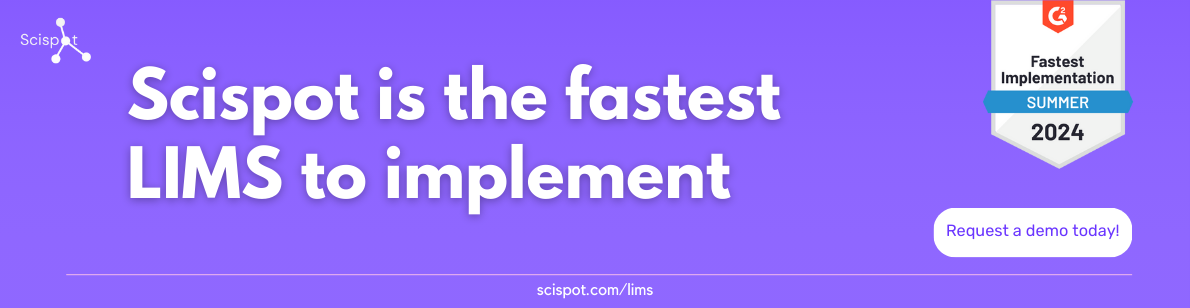
The Transformation: From Fragmented to Fluid
With Scispot OS, everything changed:
- A Knowledge Graph for Full Traceability – The teams now had a complete chain of custody from peptide design to manufacturing, ensuring data was never lost or misinterpreted.
- Automated QC Checks for Reproducibility – Standard deviation and error detection became automated, reducing experimental inconsistencies.
- APIs to Automate Protocols and Data Sharing – The bioinformatics team could now programmatically create and link research protocols, eliminating slow manual requests.
- Standardized Instrument Data Formats – LCMS, Orbitrap, and Quadrupole instruments now fed directly into a unified system, automatically formatted and linked to metadata.
The Results: A Before-and-After Transformation
✅ Then: Research requests took days to be processed manually.
✅ Now: Programmatic automation reduced request times by 40%.
✅ Then: LCMS results were scattered across multiple tools.
✅ Now: Instrument data is automatically formatted and linked to metadata.
✅ Then: Teams worked in silos, leading to miscommunications and delays.
✅ Now: A unified system keeps manufacturing, proteomics, and bioinformatics in sync.
The Takeaway: AI Should Power Science, Not Slow It Down
The real question is: how much time is your team losing to disconnected data? AI should be accelerating research—not creating roadblocks. If you’re ready to turn fragmented workflows into seamless innovation, it’s time to make your data AI-ready with Scispot OS.
