Introduction
Artificial Intelligence (AI) is transforming the biotechnology industry, offering innovative solutions to accelerate research and development (R&D). By leveraging AI, biotech firms can automate routine tasks, integrate diverse data sources, and derive actionable insights, ultimately speeding up discovery and improving experimental outcomes. This whitepaper explores the various applications of AI in biotech, detailing the challenges faced by the industry and how AI can address them.
The Need for AI in Biotech
The biotechnology sector generates vast amounts of data from experiments, clinical trials, and genomics. Traditional data management and analysis methods often fall short in handling this volume and complexity. AI in biotech provides powerful computational capabilities and sophisticated algorithms that can process and analyze large datasets quickly and accurately. For instance, AI enhances drug discovery by identifying potential drug candidates through pattern recognition and predictive modeling, significantly speeding up the R&D process.
Current Challenges in Biotech R&D
Biotech research faces several specific challenges:
- Real-time Access to Data: Data is often stored in disparate systems, making comprehensive access and analysis difficult. AI in biotech solves this by integrating and standardizing data from multiple sources, connecting users with their data and enabling them to ask contextual questions.
- Inefficient Workflows: Manual processes are time-consuming and prone to errors, reducing overall productivity. AI can automate these tasks, increasing efficiency and accuracy.
- Data Quality and Standardization: Inconsistent data formats and annotations complicate data analysis and interpretation. AI helps standardize and clean data, making it more usable.
- Regulatory Compliance: Meeting regulatory standards for data integrity and reporting requires meticulous documentation and validation processes. AI can streamline these tasks, ensuring compliance.
The most significant challenge is the lack of clean, aggregated data that can be seamlessly connected with AI. Scispot addresses this issue by integrating and standardizing data from multiple sources, making it accessible and usable for AI applications. Generative AI in biotech also plays a crucial role in improving data quality and creating predictive models. Addressing these challenges is crucial for improving the efficiency and effectiveness of biotech R&D.
AI Applications Every Biotech Should Leverage
Report Generation
AI in biotech can automate the creation of detailed and customizable reports essential for regulatory compliance, collaborative research, and external communications. These reports can include visual elements such as graphs and charts, summarizing experimental results and data trends. AI-generated reports are valuable for:
Regulatory Authorities
Supporting filing and auditing with comprehensive and accurate data summaries that meet regulatory standards, such as SEND (Standard for Exchange of Nonclinical Data) reports, Clinical Trial Reports, Investigational New Drug (IND) Applications, and New Drug Applications (NDA)/Biologics License Applications (BLA).
- SEND Reports: Essential for nonclinical data exchange, involving extensive data collection, analysis, and standardization, costing upwards of $100,000 per study.
- Clinical Trial Reports: Comprehensive reports required for each phase of clinical trials, costing between $1.5 million to $6 million per study phase.
- IND Applications: Detailed documents including preclinical study data, manufacturing information, and clinical trial plans, costing between $1 million to $2 million.
- NDA/BLA Applications: Extensive documentation compiling all clinical and nonclinical data for market approval, costing between $2 million to $5 million.
Pharma Partners (Sponsors)
Biotech companies often collaborate with pharmaceutical companies to advance drug development projects. These partnerships typically involve shared milestones, including:
- Preclinical Study Results: Reports on toxicity, efficacy, and safety data from animal studies.
- Clinical Trial Progress: Updates on patient recruitment, trial phase progress, and interim results.
- Regulatory Submission Status: Information on the submission and review status of regulatory documents.
Specific reports needed include:
- Progress Reports: Summarizing experimental outcomes, milestones achieved, and any deviations from the planned timeline.
- Efficacy Reports: Detailing results from assays and studies, such as zebrafish or non-human primate studies.
- Safety Reports: Highlighting toxicity findings from animal studies and any adverse events noted.
- Financial Reports: Providing a breakdown of the funding used, money spent, and cost estimates for upcoming phases.
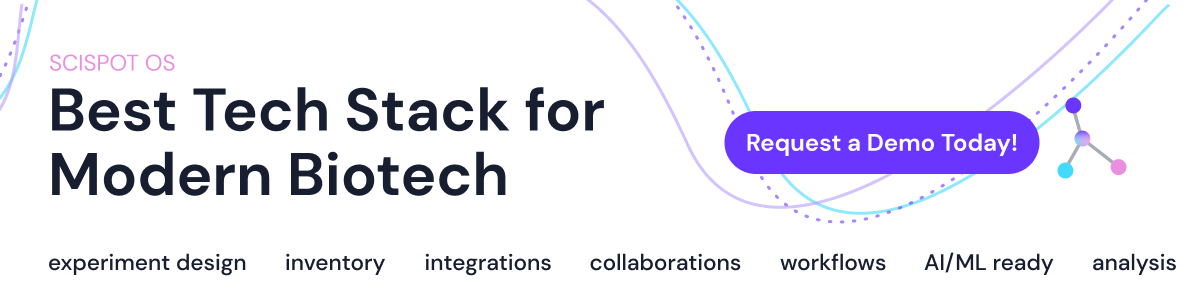
CRO Reports for Biotech Customers
Contract Research Organizations (CROs) provide essential services to biotech companies, especially in conducting in vivo studies. AI in biotech can help generate precise and timely reports for their clients, enhancing transparency and collaboration. Key types of reports include:
- Toxicity Reports: Detailed findings from animal studies (e.g., mice, rats) regarding the safety and adverse effects of new compounds. AI will utilize data from lab instruments, experimental records, and historical datasets to generate these reports.
- Efficacy Reports: Results from various assays and in vivo studies demonstrating the effectiveness of a compound. AI aggregates data from multiple sources, including assay results and observational data.
- Study Progress Reports: Regular updates on the status of ongoing studies, including any interim results and timeline projections. AI tracks milestones and integrates data from experimental logs and timelines.
- Regulatory Compliance Reports: Documentation required to meet Good Laboratory Practice (GLP), Good Clinical Practice (GCP), and other regulatory standards. AI ensures compliance by compiling data from validated sources and standard operating procedures.
- Investor Reports: Summaries of key experiments, financial metrics, and milestones for potential investors or stakeholders. AI creates these reports by analyzing project progress, financial data, and experimental outcomes.
- Grant Application Progress Reports: Detailed updates required for grant agencies like the NIH, showcasing progress and future plans. AI uses data from project management tools and experimental results to compile these reports.
By leveraging generative AI in biotech, companies can ensure accurate, comprehensive, and timely reporting, which is critical for regulatory compliance, effective collaboration, and informed decision-making.
Data Cleanup (Readiness)
AI-driven tools can clean and standardize data from various sources, ensuring consistency and accuracy. This data readiness is crucial for high-quality analysis and reliable results. In a biopharma company, essential data cleanup involves:
- Discrepancy Identification: AI in biotech can identify and correct discrepancies in data entries, such as inconsistent formats and missing values.
- Data Harmonization: AI harmonizes data from different sources to ensure uniformity, making it easier to analyze and interpret.
- Outlier Detection: Generative AI in biotech flags outliers for review, ensuring that only high-quality data is used for analysis.
Extracting Real-Time Insights
AI enables real-time data analysis, allowing researchers to extract insights and make informed decisions quickly. This capability is particularly beneficial in dynamic experimental environments where timely adjustments can significantly impact outcomes. For example, AI in biotech allows biopharma scientists to:
- Analyze Experiment Data: AI can analyze ongoing experiment data stored across multiple databases and notebooks, suggesting optimizations to improve results.
- Monitor Progress: Generative AI in biotech provides real-time updates on experiment progress, helping scientists to track milestones and adjust protocols as needed.
- Identify Trends: AI identifies trends and patterns in the data, offering insights into experimental outcomes and potential areas for further investigation.
Identifying Drug Hits Faster
AI can accelerate drug discovery by identifying potential drug candidates through advanced data integration and predictive modeling. By analyzing vast datasets from various experiments and studies, AI in biotech can highlight promising compounds, thereby speeding up the identification of viable drug hits. This fail-fast approach allows researchers to focus resources on the most promising candidates. Generative AI in biotech further enhances this process by creating novel compounds and optimizing existing ones.
Advanced Data Integration and Analysis
AI integrates seamlessly with a variety of systems and instruments, such as high-throughput screening instruments, mass spectrometry, and genomic sequencers. This capability allows for comprehensive data integration from multiple sources, providing a holistic view of experimental data. Wet lab scientists can perform sophisticated analytics without needing computational expertise or bioinformaticians, using natural language processing to execute tasks such as:
- Linear Regression: Conducting linear regression analyses to understand relationships between variables.
- Correlation Analysis: Identifying correlations between different datasets to uncover new insights.
- Predictive Modeling: Building predictive models to forecast experimental outcomes and identify potential drug candidates using generative AI in biotech.
Enhanced Data Management
AI-driven analytics facilitate the processing of complex datasets, enabling advanced analyses such as pharmacokinetic (PK) and pharmacodynamic (PD) modeling. AI in biotech can automatically perform dose-response analysis, generating precise curves that help in determining the efficacy of new compounds. This capability is essential for drug discovery, where understanding the relationship between drug dosage and biological response is critical.
AI-Assisted Lab Management
Efficient Resource Management: AI assists in tracking instrument usage, monitoring inventory levels, and detecting anomalies, ensuring optimal lab operations. For example, AI in biotech can notify lab managers when reagents are running low or when an instrument requires maintenance, preventing interruptions in research activities and ensuring continuous operation. This proactive approach to resource management enhances lab efficiency and reduces operational costs.
Proactive Maintenance: By analyzing usage patterns and identifying anomalies, AI enables proactive maintenance of lab equipment. This predictive capability helps extend the lifespan of expensive instruments and reduces downtime. For instance, generative AI in biotech can alert technicians to potential issues with a high-performance liquid chromatography (HPLC) system before they lead to significant problems, ensuring timely interventions. This capability not only improves equipment reliability but also enhances the overall productivity of the lab.
Automated Activity Summarization
AI can generate summaries of lab activities over various periods, such as daily, weekly, monthly, or quarterly. This capability provides a high-level view of lab efficiency and output, helping researchers and managers track progress and identify areas for improvement. Examples of these summaries include:
Key Updates:
- Daily Summaries: Highlighting the most recent activities, such as new lab entries (e.g., samples, inventory), protocol changes, and inventory updates.
- Weekly Summaries: Summarizing key achievements and challenges faced during the week, including detailed progress on ongoing experiments.
- Monthly Summaries: Providing a comprehensive overview of the lab's activities, including significant milestones, completed tasks, and pending issues.
- Quarterly Summaries: Offering an in-depth analysis of the lab's performance over the quarter, including trends, areas of improvement, and strategic planning insights.
Most Active Users: Tracking the most active users in the lab can help identify key contributors and ensure balanced workload distribution. These reports might include:
- Top Contributors: Listing users with the highest number of activities, such as experiments conducted, data entries, and protocol updates.
- Activity Breakdown: Detailing the types of activities performed by each user, helping to understand their contributions better.
New Lab Entries and Protocol Changes: Keeping track of new entries and changes in protocols is crucial for maintaining data integrity and ensuring compliance. Reports can include:
- New Entries: Summarizing new data entries (e.g., samples, inventory), including their source, date of entry, and responsible personnel.
- Protocol Changes: Highlighting any updates or modifications to existing protocols, including reasons for changes and approvals.
Inventory Monitoring: Monitoring inventory levels and usage can help manage resources efficiently. Reports can include:
- Inventory Updates: Summarizing additions, deletions, and current stock levels of key inventory items.
- Usage Patterns: Analyzing the consumption of reagents and materials, identifying trends, and forecasting future needs.
By leveraging AI in biotech, particularly generative AI in biotech, companies can ensure accurate, comprehensive, and timely reporting, which is critical for regulatory compliance, effective collaboration, and informed decision-making.
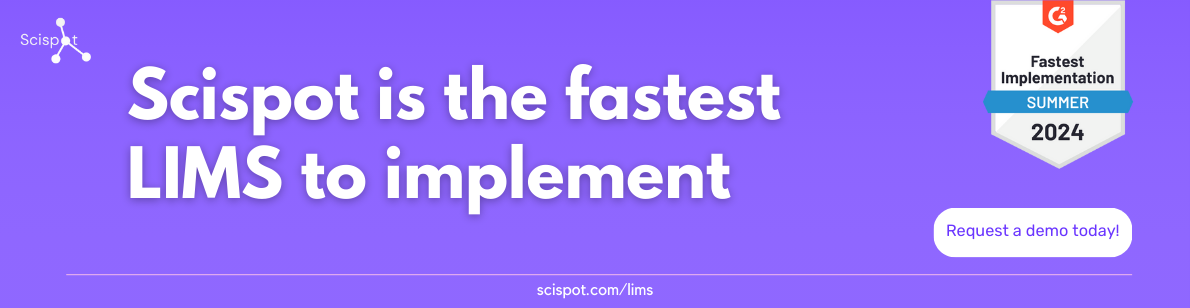
CASE STUDY: How a Biopharma Startup in South San Francisco Used Scispot AI to Enhance Small Molecule Drug Discovery
A biopharma startup successfully leveraged Scispot AI to accurately identify hits from screening data based on predefined parameters such as toxicity, IC50 values, and deviation from control measures. This approach eliminated any wait time for the wet lab team, as they no longer needed to rely on informatics teams to process the data.
Data Requirements
The project required access to the full molecule library screening data against specific cell lines or models, typically ranging from 250K to 500K molecules. Data points included compound identifiers, toxicity results, IC50 values, and control readings.
Model Inputs
- Compound Data: Specific readings for each compound (e.g., toxicity, IC50).
- Control Data: Normalized values of controls for benchmarking.
- Thresholds for Hits: Defined standards for what constitutes a hit, such as values beyond the 2nd or 3rd standard deviations from the control average.
AI Tasks
- Normalization: Scispot AI normalized compound values against controls to facilitate accurate comparisons.
- Hit Identification: Using AI in biotech, Scispot AI automatically classified compounds as hits based on the defined cutoff parameters.
- Association Studies: Leveraging generative AI in biotech, Scispot AI performed correlation analysis across multiple screens to identify common hits, patterns, or anomalies.
- Recomputation and Adjustment: Scispot AI continuously refined calculations and thresholds based on new data and feedback from scientific review.
Expected Outcomes
- Identified Hits: A comprehensive list of identified hits for each screening set.
- Statistical Analysis: Reports showing commonalities and deviations across different screens.
- Suggestions for Redefinition: Recommendations for potential redefinitions of hit criteria based on AI findings.
Implementation Steps
- Data Preparation: Cleanse and structure screening data for model ingestion.
- Model Development: Train models using supervised learning techniques with existing hit/non-hit labels.
- Validation and Iteration: Validate model predictions against known data and iterate based on scientific feedback.
- Integration: Integrate AI solutions with existing data systems for real-time analysis and reporting.
Performance Metrics
- Accuracy: High accuracy of hit predictions.
- Relevance: Significant relevance of identified patterns across screens.
- Efficiency: Improved computational efficiency in handling large datasets.
Documentation and Reporting
- Performance Logs: Maintain a detailed log of model performance metrics, errors, and adjustments.
- Regular Updates: Provide regular updates and reports to stakeholders outlining findings, recommendations, and areas for further research.
By integrating Scispot AI, the biopharma startup enhanced its small molecule drug discovery process. AI in biotech enabled the company to rapidly and accurately identify potential drug candidates from extensive screening data without any wait time for the wet lab team to rely on informatics teams. Generative AI in biotech enhances the ability to find common patterns and refine hit criteria continuously. This approach not only improved the accuracy and efficiency of drug discovery but also provided actionable insights for further research and development, establishing Scispot AI as a critical tool in their success.
Outcomes and Results
The integration of AI in biotech has led to significant improvements in research efficiency, data management, and experimental outcomes. Labs utilizing AI systems report faster data processing times, reduced errors, and an enhanced ability to derive actionable insights. For example, a biotech company using generative AI in biotech for drug discovery noted a 30% increase in the identification of viable drug candidates due to improved data integration and analysis. Automation of routine tasks has freed researchers to focus on more complex and creative aspects of their work, driving innovation and accelerating the development of new therapies. These outcomes demonstrate the transformative impact of AI on biotech R&D, showcasing tangible benefits such as increased productivity, reduced costs, and faster time-to-market for new products.
Challenges and Considerations
Data Quality and Standardization
The effectiveness of AI in biotech heavily depends on the quality and standardization of data. Inconsistent or poorly annotated data can lead to inaccurate insights. Implementing rigorous data management practices, including standard operating procedures for data entry and maintenance, is essential to maximize the benefits of AI. Ensuring high-quality, standardized data inputs is crucial for accurate and reliable AI-driven analysis.
Ethical and Regulatory Compliance
The effectiveness of AI in biotech heavily depends on the quality and standardization of data. Inconsistent or poorly annotated data can lead to inaccurate insights. Implementing rigorous data management practices, including standard operating procedures for data entry and maintenance, is essential to maximize the benefits of AI. Ensuring high-quality, standardized data inputs is crucial for accurate and reliable AI-driven analysis.
Conclusion
AI in biotech is set to transform research and development by improving experimental design, data management, and decision-making. By automating routine tasks, integrating diverse data sources, and providing advanced analytical capabilities, AI enables researchers to focus on innovation and discovery. For instance, generative AI in biotech can streamline the drug discovery process, leading to more efficient identification of viable candidates.
However, realizing the full potential of AI in biotech requires addressing challenges related to data quality, ethical considerations, and regulatory compliance. Ensuring high-quality, standardized data inputs and maintaining transparency in AI decision-making processes are crucial. Additionally, complying with ethical standards and regulatory guidelines, such as those set by the FDA, is essential to gain trust and ensure reliable outcomes.
As AI technologies continue to evolve, their integration into biotech will drive significant advancements in scientific research and development. Scispot is at the forefront of this transformation, offering AI-powered solutions that enhance data integration, automate routine tasks, and provide real-time insights. By partnering with Scispot, labs can leverage the latest advancements in AI to accelerate their research, reduce costs, and bring new therapies to market faster. Scispot is committed to integrating AI with biotech R&D, one lab at a time, ensuring that researchers have the tools they need to succeed in this rapidly evolving field.
