Over the last decade, discussions have centered on building tools that are suited for either hands-on lab work or computational analysis. However, with the rise of AI, this debate is becoming less relevant. AI is enabling both wet lab and dry lab professionals to work together more seamlessly and efficiently, eliminating many traditional barriers.
Generative AI’s true potential in life sciences lies not in simple automation but in its ability to bridge the gap between wet lab and dry lab environments. By enhancing communication, integrating lab systems, and automating workflows, AI helps scientists in both domains collaborate more effectively.
What are Wet Labs and Dry Labs?
Before delving into the integration of wet lab and dry lab environments, it’s essential to clarify their roles.
Wet labs are primarily focused on conducting hands-on experiments, such as chemical reactions, biological assays, and other processes involving physical manipulation of samples. These labs deal with physical substances, including liquids, solids, and gases, and researchers here are skilled in managing these materials and observing their reactions.
Dry labs, on the other hand, are more computational and analytical in nature. These labs focus on analyzing data, building models, and using computer simulations. Dry labs don’t deal with physical substances directly but instead work with data collected from experimental procedures.
However, the distinction between wet and dry labs is becoming increasingly blurred. Many wet labs now integrate computational tools for data analysis, while dry labs are incorporating experimental elements like simulations to test hypotheses. The real challenge is enabling seamless collaboration between these two environments to streamline workflows and enhance productivity.
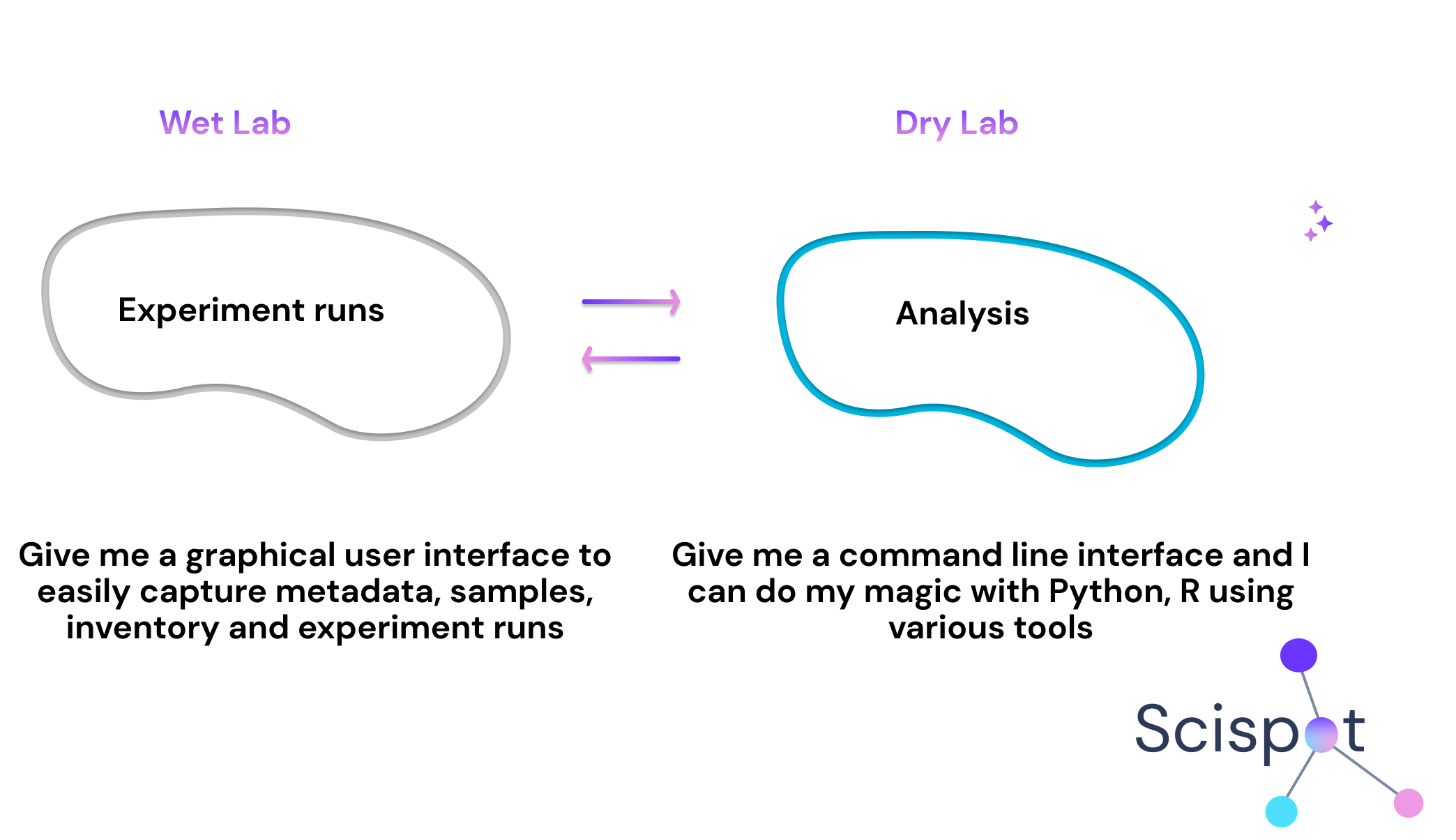
The Gap Between Wet and Dry Lab
The gap between wet lab and dry lab workflows often results in inefficiencies. Assay researchers in the wet lab manage experiments and physical testing, while dry lab professionals focus on analyzing the data generated from these experiments. The challenge arises when these two groups are forced to work with different tools, languages, and methodologies, which can create friction in communication, slow down research timelines, and cause errors.
In many traditional setups, wet lab scientists rely on dry lab teams for downstream data analysis. However, the lack of shared understanding between these groups, along with the tools they use, can complicate the workflow. Wet lab scientists may lack familiarity with the complexities of data analysis software, while dry lab experts may not fully grasp the experimental context that influences the data.
Challenges in Laboratory Workflow Automation
Several challenges arise when trying to automate workflows between wet labs and dry labs:
- Technical Differences: Wet lab experts excel at planning experiments, preparing samples, and conducting hands-on tests. Their focus is on practical, real-world changes that happen during the experiment. On the other hand, dry lab professionals focus on analyzing large datasets, building models, and running simulations on computers. Their work requires different skills and tools, which makes communication and integration difficult.
- Lack of Shared Understanding: The “handshake” problem emerges when wet lab researchers and dry lab scientists operate with different goals and tools. Data may not easily transfer between systems, and critical nuances—such as the conditions under which data was collected—are often lost in translation. This makes it difficult to analyze data accurately and integrate findings into the broader research.
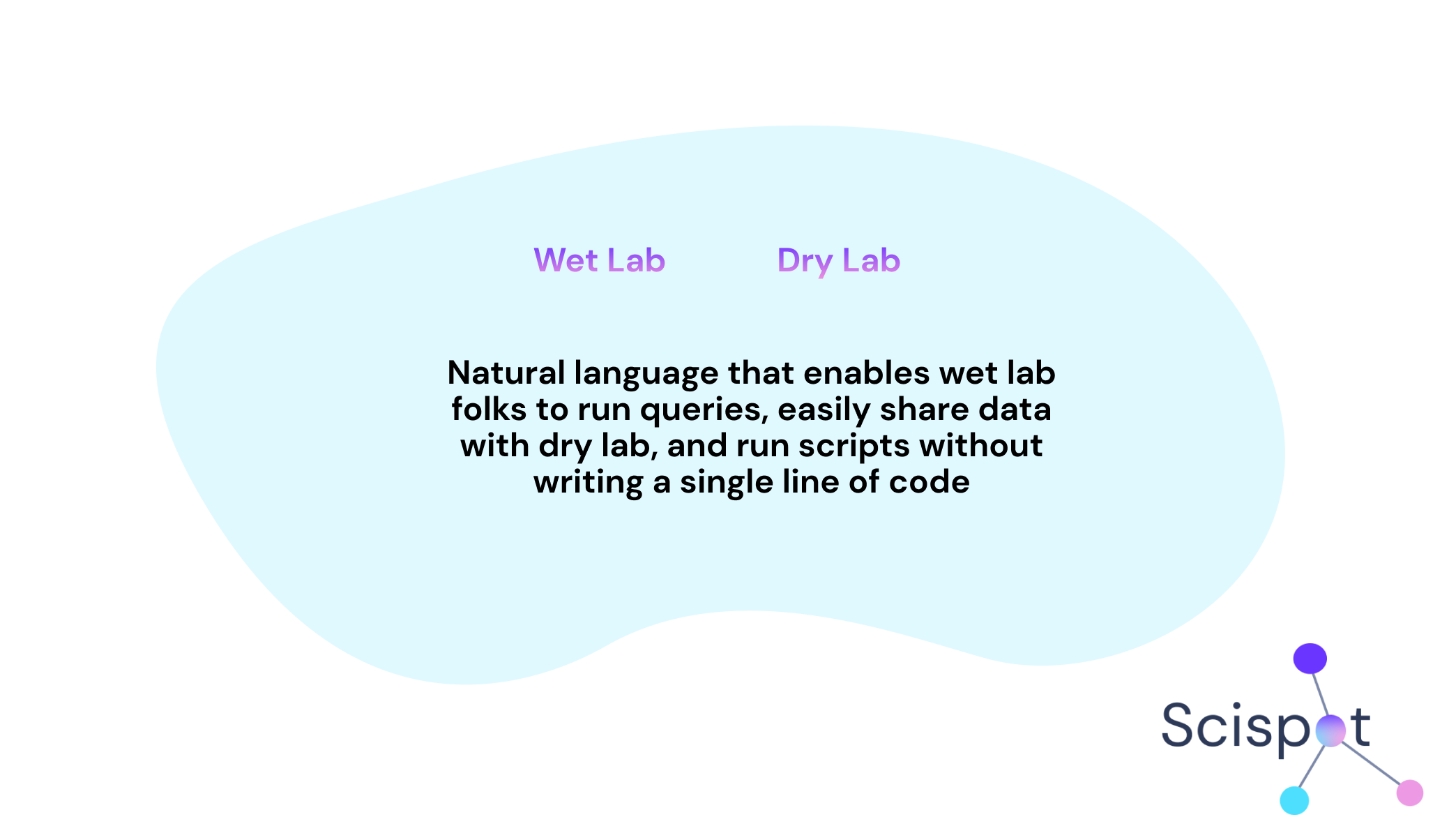
The Role of AI in Bridging Wet and Dry Labs
AI has the potential to significantly reduce the gap between wet lab and dry lab environments. By using AI-powered tools, wet lab researchers can now automate repetitive tasks such as running scripts for sample management or analyzing experimental data. This automation not only saves time but also reduces human error.
For example, wet lab professionals can now interact with lab systems through natural language processing (NLP), allowing them to execute complex commands and analyses without needing advanced computational skills. This creates a bridge between the hands-on work of the wet lab and the data-heavy processes in the dry lab.
Generative AI has already shown great promise in integrating different systems. By automating data transfers, simplifying data analysis, and facilitating communication between wet lab and dry lab teams, AI tools can make the overall workflow more efficient. AI also helps by providing insights and actionable data at faster speeds, which accelerates the research process.
Lab Integration Software and Workflow Automation
Lab integration software and workflow automation tools promise to alleviate many of the issues caused by the divide between wet and dry labs. However, these tools often fail to fully address the differences in operational goals and technical capabilities between the two environments.
Many wet lab researchers find that lab automation software is too complex or doesn’t fit into their experimental workflows, while dry lab professionals may feel that these tools lack the computational depth needed for sophisticated analysis. The key challenge is developing tools that work for both teams by accommodating their different skill sets, terminologies, and objectives.
The Power of Natural Language for Lab Integration
By incorporating natural language processing (NLP) into lab systems, AI can make the interface between wet and dry labs more user-friendly. Wet lab scientists can now use simple language to run scripts and execute commands for sample management or analysis, without requiring deep technical knowledge. At the same time, dry lab teams can interact with the system to process and analyze data more intuitively.
Scispot is leading the way by implementing AI-powered tools that help break down the barriers between wet and dry labs. Our AI Assist features enable seamless communication between wet lab researchers and dry lab professionals by integrating wet lab research and computational analysis into a unified workflow. Whether it’s sample management, data analysis, or collaboration through APIs, Scispot is paving the way for the next generation of lab integration.

AI Assist Features by Scispot
Our AI Assist features provide wet lab professionals with powerful tools to automate and streamline their workflows using natural language commands. These tools allow for easy integration with Scispot’s API and Python scripts, making it simpler for scientists to execute complex commands without extensive coding skills.
Scispot is working with labs in North Carolina and San Francisco to refine our AI-powered solutions. These tools are already making a real impact by reducing friction between wet lab and dry lab workflows and improving efficiency across the board.
Conclusion
By bridging the gap between wet lab and dry lab environments, AI is transforming how scientific research is conducted. The future of lab workflow management involves seamless integration and collaboration between these two domains, allowing wet lab and dry lab professionals to work more effectively together. Scispot is committed to advancing this vision by providing cutting-edge AI tools that enhance communication, reduce errors, and accelerate research timelines.
Let’s combine wet labs and dry labs, simplify procedures, and enhance lab management with AI-powered solutions. The future of research is collaborative, efficient, and faster than ever before.
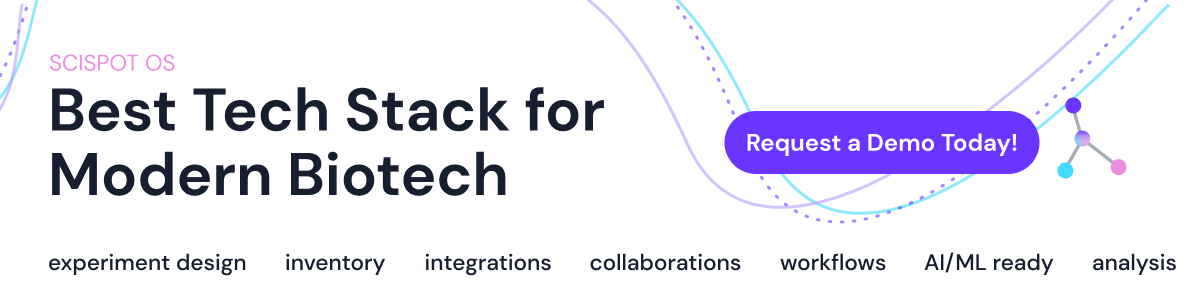